What the Machine Learning Element of Big Data Has to Offer the Retail Industry
What is machine learning? Machine learning (often shortened within the industry as ML) is a kind of artificial intelligence (AI), which is an offshoot of big data. AI, and by extension, ML, utilize big data in a different way than typical analysis. These practices are capable of taking in data, building assumptions based on the data, testing hypotheses about those assumptions, and drawing conclusions from the results. Though not nearly as complex and sophisticated as human learning, AI and ML can do rudimentary logic by themselves. Yes, it sounds a little creepy (and potentially even 2001 Space Odyessy-ish), but there are numerous practical uses for AI and ML. What can ML do for retailers?
Machine Learning Can Help Make Product Recommendations
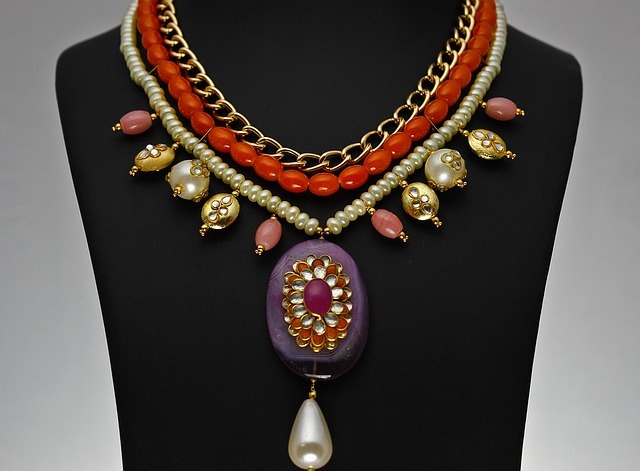
Recommending another necklace to a shopper already buying a necklace might not be nearly as effective as offering her a handbag or hair accessories. ML makes sure that your recommendations get better every time someone shops with you.
Buyers typically buy certain things together. While some of these are basic and require no real analysis (buying AA batteries with the order of a computer mouse or a bookmark with an order of best sellers), but others are less obvious and difficult to ascertain without analysis. With machine learning, these recommendations can even improve over time. The program can learn when its guesses were right or wrong and alter its recommendations for next time.
Machine Learning Can Improve A/B Testing
Machine learning can take your vital A/B testing to whole new heights. First, the programs can handle much more than just two variables. These programs can also detect hidden correlations and make improvements to many retailers’ processes over time. You can use ML to improve store layouts, checkout processes, refine landing pages, develop better online ads, and much more.
Machine Learning Can Help Stop Fraud
The biggest threat to today’s retailers is cybercrime and retail fraud. No business wants to end up like Target, Michael’s, and Home Depot after their headline news data breaches. Machine learning can ‘learn’ to assess normal patterns of consumer behavior and detect anomalies in those patterns that can indicate fraud. This could save billions of dollars per year in lost revenue, legal costs, and public relations fixes.
Machine Learning Can Help Optimize Your Pricing Structures
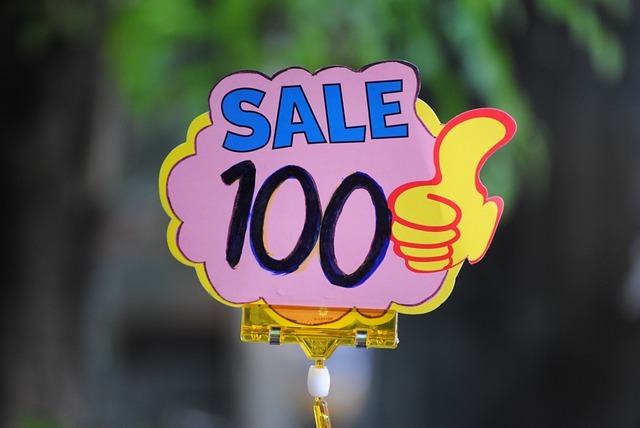
How much can you charge before the extra profits aren’t worth the loss in sales? ML gets better and better at this, and can even learn to make predictions about future pricing based on the upturn or downturn of the economy and other factors.
Pricing structures are always tricky, because as soon as you get a handle on it, things change and everything is different. What consumers are willing to pay for a high-end watch or mid-range computer when times are good is not what they’re willing to cough up when the economy hits the skids. ML can be used not just to find a good pricing setup for any given time, but to predict changes that could affect pricing down the road and make adjustments before the current prices get out of whack.
Machine Learning Can Improve Your Inventory Forecasting
Another tricky issue for retailers is forecasting inventory. ML can help predict trends, including higher than usual or lower than usual demand, so that you can always have enough (but never too much) stock on hand. ML can also help you order enough of the hottest colors for next season, get rid of excess inventory by identifying the best markets for offloading overstock, and even determine any supplier issues that might affect your inventory planning.
Are you a retailer in need of more information on how big data can improve your efforts? If so, Big Data Week is your kind of gig. See the full speaker lineup here.