Ready for a Swim? How to Tell When You’re Ready for a Data Lake (or If There’s a Better Solution)
As most organizational data sets grow beyond the capabilities of the traditional data warehouse, a lot of businesses are taking a look at the option of building a data warehouse. But scanning the tech news headlines and IT blogs, you’ll find two camps: the one saying that the data lake is the salvation of your data architecture and data plans, and the camp that maintains a data lake is nothing but a data swamp — the place where good data goes to die without a decent burial.
The truth lies somewhere in the middle. Yes, a data lake can solve a lot of problems (for one, it’s a whole lot cheaper), but no, it isn’t the end-all solution for storing unlimited quantities of unstructured data. It has to be built correctly and managed properly to be useful. Without some solid maintenance (such as assuring that all of the data is well-endowed with rich and useful metadata), it does become a bit swampish. Here’s how to tell if a data lake is right for you, and what to do if you decide that is or isn’t the best option.
Is the Current Data Warehouse Solution Insufficient?
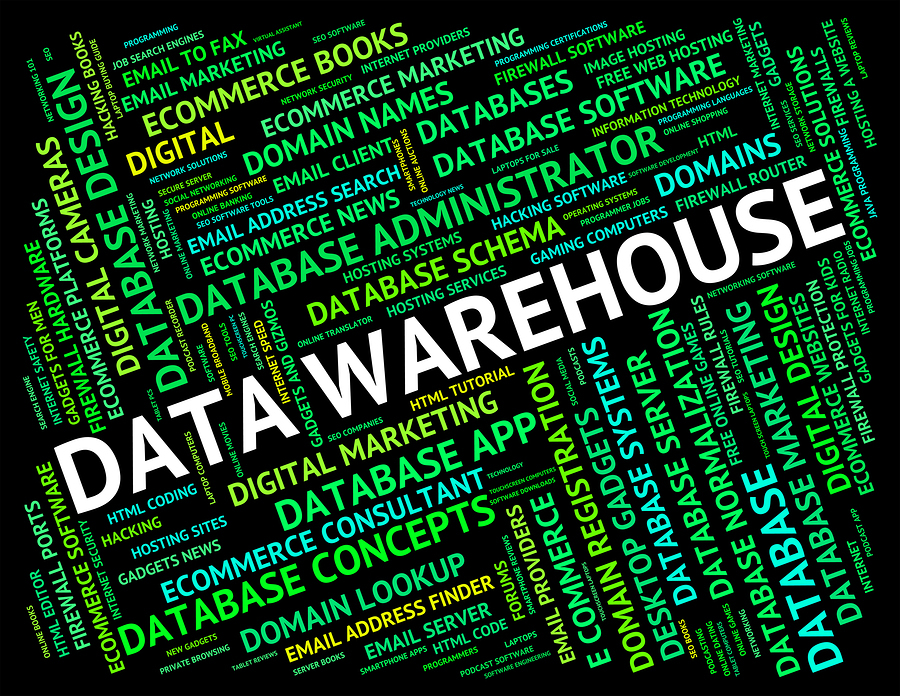
The data warehouse usually becomes insufficient when the organization finds a need to collect large stores of unstructured data.
The first heads-up that you need a data lake is when you find yourself with an insufficient data warehouse, or worse, end up with multiple data warehouses or data stores. A data lake is primarily different from a data warehouse in that you can store the data in schemata-less, raw format. The second differentiation is that it can get quite big and sprawling without running up a hefty tab. You can build a data lake in Hadoop, Spark, or with a combination of the two. In fact, for all the arguments over Spark versus Hadoop, the two actually share a sandbox rather well.
Do You Need a Cheaper Way to Store Both Raw and Managed Data?
Traditional storage is coming down in price, but for enterprise quantities of data, that price is still a bit eye-popping. In an era in which IT is supposed to do it all, but still manage to keep prices at the bargain-basement level, the data lake allows you to collect and house data from numerous in-house stores, plus your external sources (like social media) and still keep the old IT budget within reason. If you opt for a cloud-based big data solution, you scan swap that data storage bill from the CapEx expense ledger to the OpEx ledger, thereby eliminating some of the hoops you have to jump through to get charges approved.
Are You Trying to Eliminate Data Silos Around the Organization?
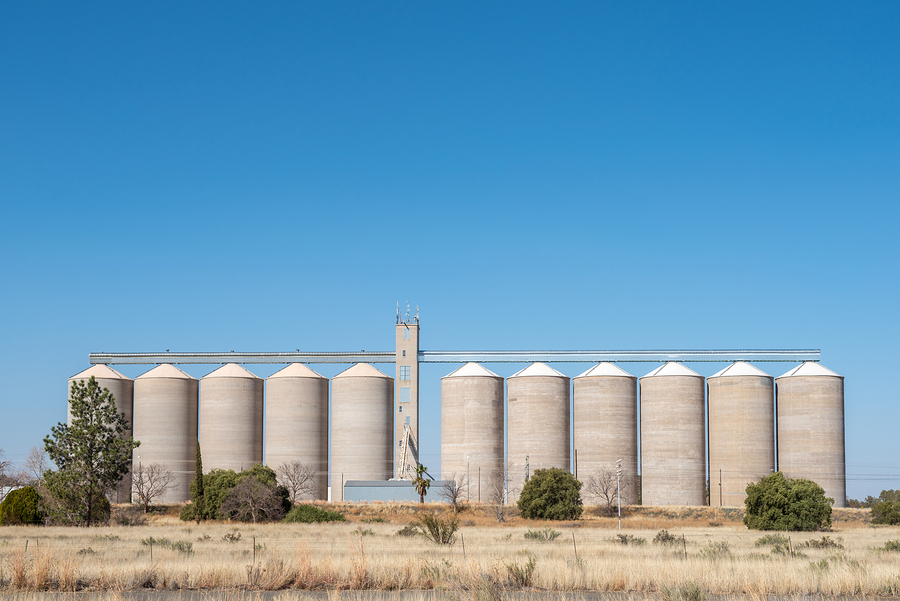
As long as the organization holds on to data silos, a comprehensive data analytics plan is not possible. The data has to be consolidated, and the data lake is ideal for that.
One of the most practical and powerful uses for a data lake is to collect large stores of data from all of your in-house sources (the operating systems, applications, mobile apps and/or IoT devices, existing data warehouse(s), etc.), plus all of the data you want to bring in from outside sources. The data lake doesn’t even require that you know what you’re going to do with the data yet, because it stores the data in its native format, ready for conversion at a later date. It can manage all of your structured data, plus the semi-structured and unstructured stuff, so that when the big bright light goes off over your analytics team’s head, the data they need to produce sheer genius is right there waiting.
Is the data lake right for you? If so, you’ll want to be sure that it’s built and maintained wisely, so that all of the data is easily retrievable when the time comes. You can learn a lot about the data lake, as well as the alternatives you have, at a Big Data Week event near you. See the full speaker lineup here.